Paper reading: Efficient Estimation of Word Representations
From google brain: https://arxiv.org/pdf/1301.3781.pdf
projection layer: 投射层,maps word index to continuous vector space. ("hidden layer is removed")
projection layer input 可能是一个one hot encoding 的 vector, 就是第i个word,除了位置i是1,其他全是0。这个层没有softmax, 只是单纯的投射过程。
http://mi.eng.cam.ac.uk/~gwb24/publications/mphil.thesis.pdf
之前的 forward neural network, 用前面的词来预测这个词w(t)
Bag of words: 通过周围的词 (context),预测这个词 w(t)。不仅之前的词,被用到,之后的词也被用到。当然这个之前之后是有限制的 (time step)。
Skip gram: 通过这个词 w(t), 预测周围的词
最开始的input 应该都是one hot encoding value
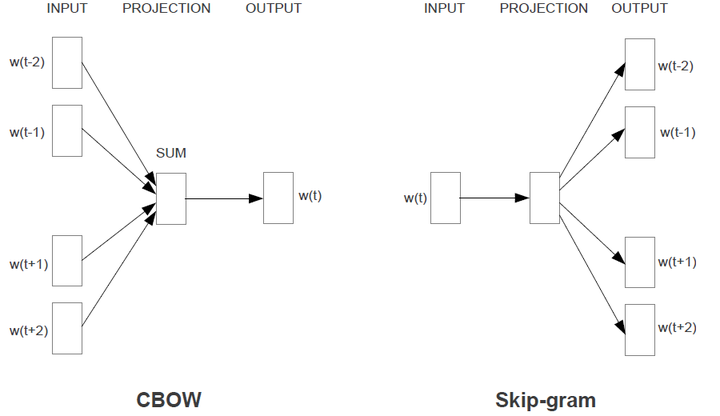
最后的word vector 应该就是这个projection layer对应的weight, 每个词都会有一个N dimensional vector 表示,这个N可以是自定义的
比如2D result
Library
projection layer: 投射层,maps word index to continuous vector space. ("hidden layer is removed")
projection layer input 可能是一个one hot encoding 的 vector, 就是第i个word,除了位置i是1,其他全是0。这个层没有softmax, 只是单纯的投射过程。
http://mi.eng.cam.ac.uk/~gwb24/publications/mphil.thesis.pdf
之前的 forward neural network, 用前面的词来预测这个词w(t)
Bag of words: 通过周围的词 (context),预测这个词 w(t)。不仅之前的词,被用到,之后的词也被用到。当然这个之前之后是有限制的 (time step)。
Skip gram: 通过这个词 w(t), 预测周围的词
最开始的input 应该都是one hot encoding value
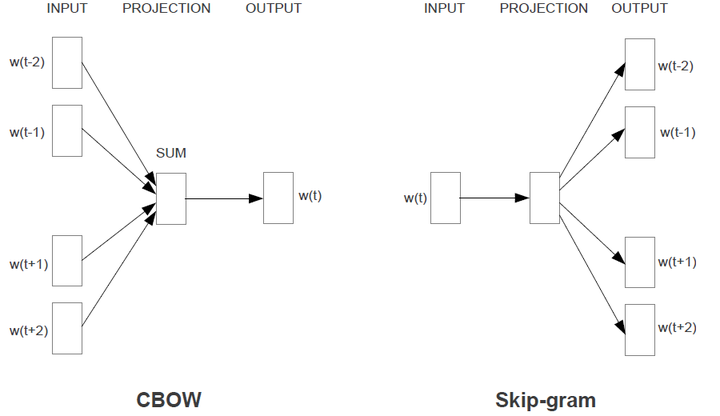
最后的word vector 应该就是这个projection layer对应的weight, 每个词都会有一个N dimensional vector 表示,这个N可以是自定义的
比如2D result
Library
- gensim
- https://radimrehurek.com/gensim/models/word2vec.html
- N 用size 来表示
- sg=1 如果想用skip gram
- Tensorflow word2vec:
- https://www.tensorflow.org/tutorials/word2vec
评论